Sampling, Central Limit Theorem, & Standard Error
Building Statistical Foundations: From Sampling Techniques to Informed Inferences
4.71 (7 reviews)
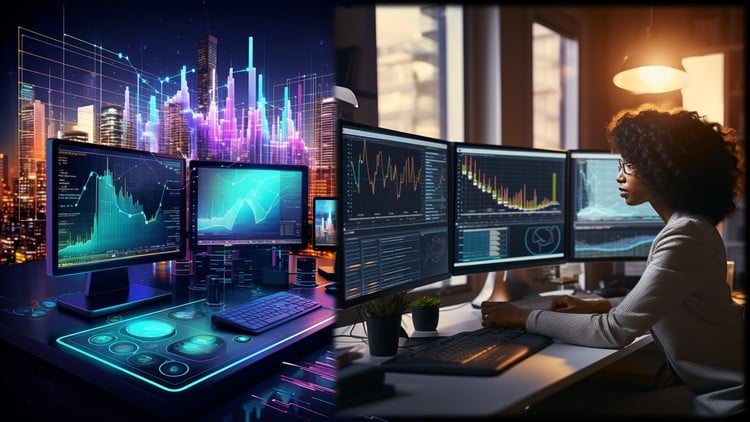
4,077
students
4.5 hours
content
Nov 2024
last update
$44.99
regular price
What you will learn
Define key statistical terms, including population, sample, parameter, and statistic, to build a foundation in statistical language and concepts.
Identify and differentiate between various sampling methods, such as simple random sampling, stratified sampling, and cluster sampling.
Illustrate the concept of sampling bias and explain strategies to minimize sampling error, enhancing the validity of sample-based conclusions.
Describe the Central Limit Theorem and explain its significance in enabling normal approximation for sample means, regardless of the population distribution.
Calculate standard error and analyze how sample size influences the precision of sample statistics.
Evaluate the representativeness of samples in real-world applications and assess the implications of sample variability on inferential accuracy.
Integrate sampling methods, the CLT, and standard error to form a coherent approach to statistical inference in various applied fields.
Justify statistical conclusions drawn from sample data and reflect on the role of inferential statistics in research and decision-making.
6141147
udemy ID
8/22/2024
course created date
12/10/2024
course indexed date
Bot
course submited by